Please note: This course will be taught in hybrid mode. Hybrid delivery of courses will include synchronous live sessions during which on campus and online students will be taught simultaneously.
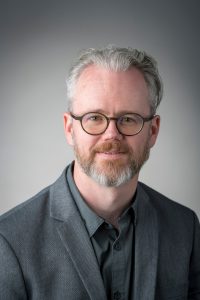
Mark Pickup is a professor in the Department of Political Science at Simon Fraser University. He is a specialist in Political Behaviour, Political Psychology and Political Methodology. Substantively, his research primarily falls into three areas: political identities and political decision-making; conditions of democratic responsiveness and accountability; and polls and electoral outcomes. His research focuses on political information, public opinion, political identities, norms and election campaigns within North American and European countries. His methodological interests concern the analysis of longitudinal data (time series, panel, network, etc.) with secondary interests in Bayesian analysis and survey/lab experiment design. His work has appeared in, among others, American Journal of Political Science, Journal of Politics, British Journal of Political Science, Political Analysis, Electoral Studies, Political Behavior, and Canadian Journal of Political Science. He is also the author of Introduction to Time Series in the Sage Publications, Quantitative Applications in the Social Sciences series.
Course description
Statistical models can be applied to longitudinal data. Chronological sequences of observations —time series data— allow us to examine the movement of social science variables over time (e.g., public opinion, government policy, judicial decisions, socioeconomic measures), allowing analysts to estimate relationships between variables and test hypotheses. Data collected over both units (e.g., survey respondents, states, countries) and time (e.g., days, months, years) —panel data— are common in the social sciences. By gaining leverage across units and over time, these data help us answer important questions that would be difficult if we only looked at a single point in time (e.g., cross section). Despite these advantages, longitudinal data often show forms of heterogeneity as well as temporal and spatial dependence that make standard regression approaches inappropriate.
The course will provide a comprehensive discussion of the fundamental concepts in time series analysis, including: autoregressive, moving-average and unit-root processes; trending, cycling and structural breaks; weak dependence; and the three most important assumptions underlying longitudinal estimates of models, stationarity, exogeneity and balance. It will also provide a broad understanding of panel data analysis, including: the structure and properties of panel data; spatio-temporal dependence; approaches to addressing spatial heterogeneity, such as random and fixed effects; and testing for and modeling dynamics. Throughout, we will also discuss several smaller topics in longitudinal data.
A sound background in linear regression models is assumed but prior training in longitudinal data analysis is not required. The course will make use of basic algebra. The lab component of this course will employ Stata. Some familiarity with Stata would be helpful but for those without that familiarity, labs notes will provide a brief introduction.
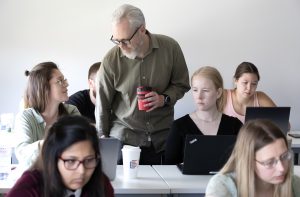
Pre-class preparation
For those that would like a mathematics refresher:
Wooldridge, Jeffrey. 2012. Introductory Econometrics: A Modern Approach. 5th ed. Mason, Ohio: Thomson/South-Western, Appendix B.1- B.4.
Gill, Jeff. 2006. Essential Mathematics for Political and Social Research. Cambridge: Cambridge University Press, 5.1-5.3
Participants will want to obtain:
Pickup, Mark. 2014. Introduction to Time Series Analysis. Quantitative Applications in the Social Sciences, (QASS) Series. Thousand Oaks, California: Sage Publications, Inc. (this will be provided by ESS)
Hsiao C. 2014. Analysis of Panel Data, 3rd Edition. New York, NY: Cambridge University Press. (this will be provided by ESS)
Background Knowledge
Maths
Calculus – moderate
Linear Regression – strong
Statistics
OLS – strong
Maximum Likelihood – elementary
Software
Stata – elementary
R – elementary

Course outline
Topic 1: A Review of Essential Mathematics and Statistical Theory
- Wooldridge, Jeffrey. 2012. Introductory Econometrics: A Modern Approach. 5th edition. South Western Educational Publishing, Chapters 2-4.
- Pickup, M. 2014. Chapter 1.
- Philips, A.Q. 2021. “Stata: A brief introduction.”
Topic 2: The Basics of Time Series analysis
- Pickup, M. 2014. Chapters 2-3.
- Pickup, M. and P. Kellstedt. 2021. “Balance as a Pre-Estimation Test for Time Series Analysis.” Political Analysis (Published Online):1-10.
Topic 3: Stationary Dynamic Time Series Models
- Pickup, M. 2014, Chapters 4-5.1 inclusive.
Topic 4: Model Selection and Nonstationarity
- Pickup, M. 2014. Chapters 5.2-6 inclusive.
- Beck, N. 1991. “Comparing Dynamic Specifications: The Case of Presidential Approval.” Political Analysis 3: 51-87
Topic 5: Panel data fundamentals, describing and summarizing panel data
- Hsiao C. 2014. Analysis of Panel Data, 3rd Edition. New York, NY: Cambridge University Press. Ch. 1.
- Beck, N. 2001. “Time-series-cross-section data: What have we learned in the past few years?” Annual Review of Political Science 4(1):271-293.
Topic 6: Identifying spatio-temporal dependence
- De Hoyos, R. E. and Sarafidis, V. (2006). “Testing for cross-sectional dependence in panel-data models.” The Stata Journal 6(4):482-496.
- Pesaran, M. H. 2021. “General diagnostic tests for cross section dependence in panels.” Empirical Economics 60: 13-50.
- Phillips, P.C. and Sul, D. 2003. “Dynamic panel estimation and homogeneity testing under cross section dependence.” The Econometrics Journal 6(1):217-259.
Topic 7: FGLS procedures for standard error corrections
- Beck, N and J. Katz. 1995. “What To Do (and Not To Do) with Time Series Cross-Section Data.” American Political Science Review 89:634-47.
- Parks, R. W. 1967. “Efficient estimation of a system of regression equations when disturbances are both serially and contemporaneously correlated.” Journal of the American Statistical Association 62(318):500-509.
Topic 8: Approaches to heterogeneity: Fixed and random effects
- Hsiao C. 2014. Analysis of Panel Data, 3rd Edition. New York, NY: Cambridge University Press pp. 31-50.
- Zhu, L. 2012. “Panel Data Analysis in Public Administration: Substantive and Statistical Considerations.” Journal of Public Administration Research and Theory 23:395-428.
- Mummolo, J. and Peterson, E. 2018. “Improving the interpretation of fixed effects regression results. Political Science Research and Methods 6(4):829-835.
- Jordan, Soren and Andrew Q. Philips. 2022. “Improving the interpretation of random effects regression results.” Political Studies Review: 1-11.
Topic 9: Alternative models for unit heterogeneity
- Hsiao C. 2014. Analysis of Panel Data, 3rd Edition. New York, NY: Cambridge University Press pp. 50-56.
- Bell, A., and Jones, K., 2015. “Explaining fixed effects: Random effects modeling of time-series cross-sectional and panel data.” Political Science Research and Methods, 3(1):133-153.
- Bell, A., M. Fairbrother and K. Jones. 2019. “Fixed and random effects models: Making an informed choice.” Quality and Quantity 53: 1051-1074.
- Schunck, R. 2013. “Within and between estimates in random-effects models: Advantages and drawbacks of correlated random effects and hybrid models.” The Stata Journal 13(1): 65-76.
Topic 10: Modeling and interpretation under dynamic models
- Hsiao C. 2014. Analysis of Panel Data, 3rd Edition. New York, NY: Cambridge University Press pp. 80-86.
- Williams, L.K., and G.D. Whitten. 2012. “But wait, there’s more! Maximizing substantive inferences from TSCS models” The Journal of Politics 74(3):685-693.
- Beck, N. and J.N. Katz. 2011. “Modeling dynamics in time-series-cross-section political economy data.” Annual Review of Political Science: 331-352Williams, L.K., and G.D. Whitten. 2011. “Dynamic simulations of autoregressive relationships.” The Stata Journal 11(4):1-12.
Topic 11: Panel unit root and cointegration testing
- Hlouskova, J. and M. Wagner. 2006. “The performance of panel unit root and stationarity tests: Results from a large scale simulation study.” Econometric Reviews 25(1):85-116.
- Maddala, G.S. and Shaowen Wu. 1999. “A comparative study of unit root tests with panel data and a new simple test.” Oxford Bulletin of Economics and Statistics, Special Issue 61(S1):631-652.
- Westerlund, J. 2005. “New simple tests for panel cointegration.” Econometric Review 24(3):297316.
- Neal, T. 2014. “Panel cointegration analysis with xtpedroni.” The Stata Journal 14(3):684692.
Making Contact
The best way to contact me is via e-mail. mark.pickup@sfu.ca